[Introduction]
Data assimilation (DA) is an objective technique that combines imperfect, incomplete and irregular observations with our knowledge of the physical laws, manifested in the form of numerical prediction models to provide regular and physically consistent estimate of the true state.
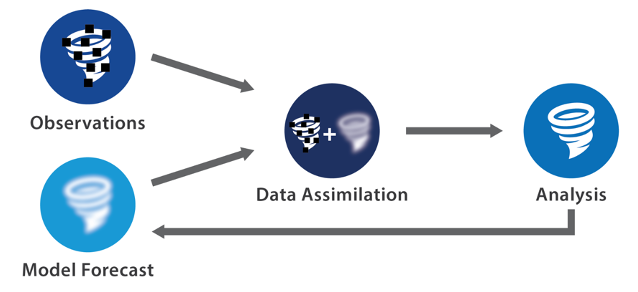
Figure adapted from METR 6313 Advance Data Assimilation lecture notes taught by Prof. Xuguang Wang and NOAA Science Advisory Board Priority for Weather Research (PWR) report (2021).
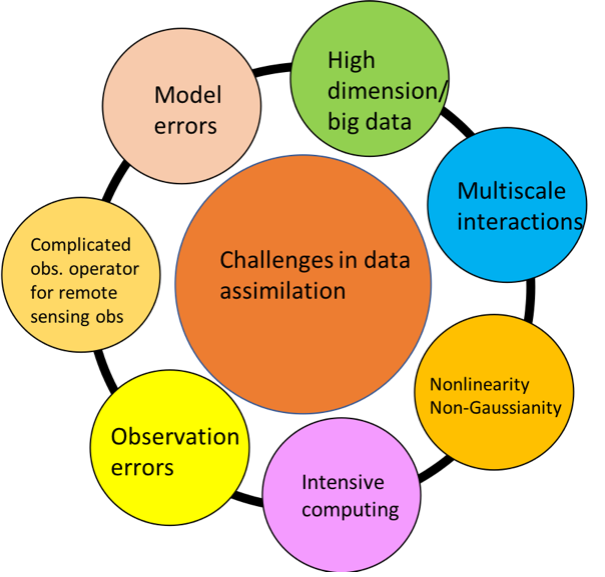
Significant scientific and technical challenges exist in data assimilation associated with the future landscape of high resolution multiscale numerical model and a myriad of high volume of existing and new observations. Therefore, innovation research and development for data assimilation are in great demand. MAP develops novel methodologies and algorithms for ensemble based, variational and hybrid ensemble-variational data assimilation.
Most recent efforts are highlighted as follows:
* ======================================================================== *
Highlights of Recent and Ongoing Research
[A new ensemble based multiscale data assimilation method: MLGETKF]
From Wang et al. (2021)
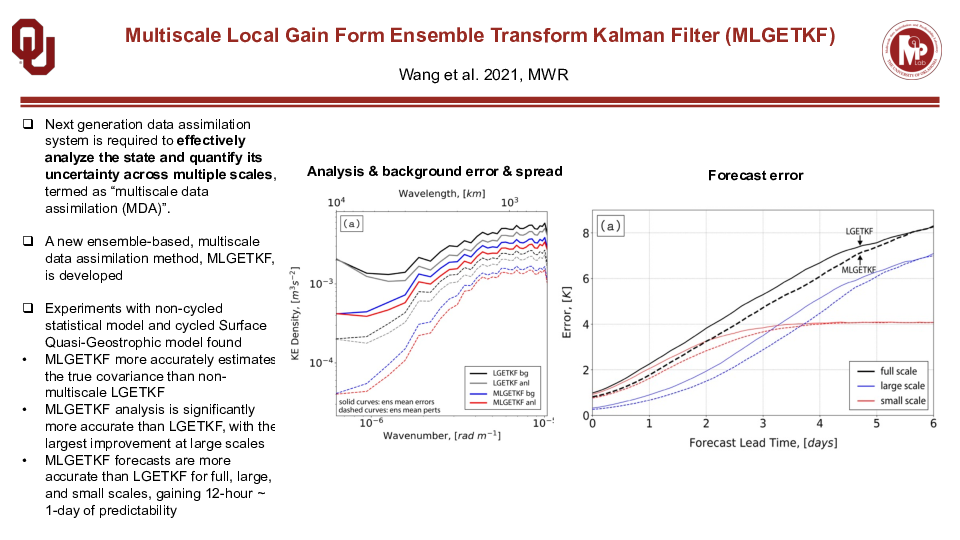
[Development of non-gaussian and non-linear filter]
From Feng, J., X. Wang et al. (2020)
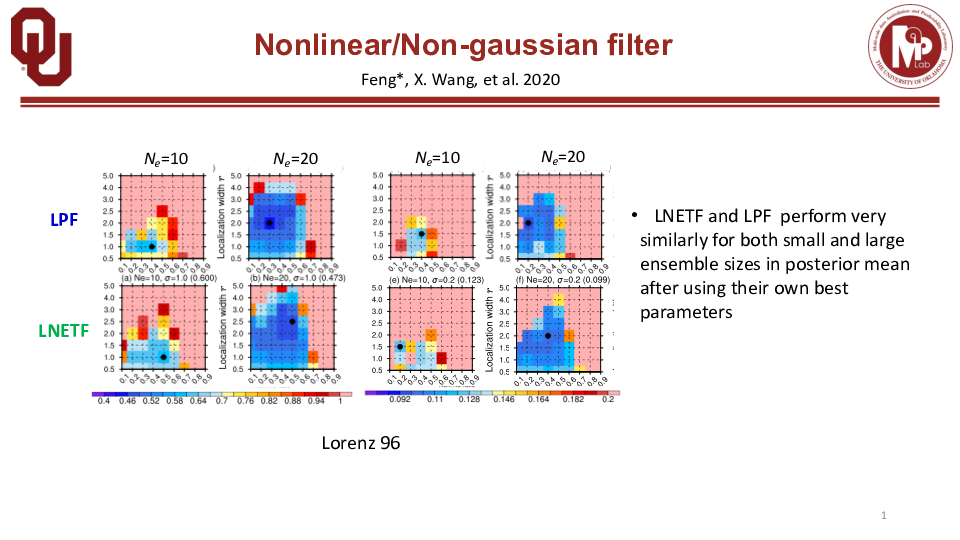
[Scale dependent and variable dependent covariance localization]
From Wang and Wang (2022; In Review)
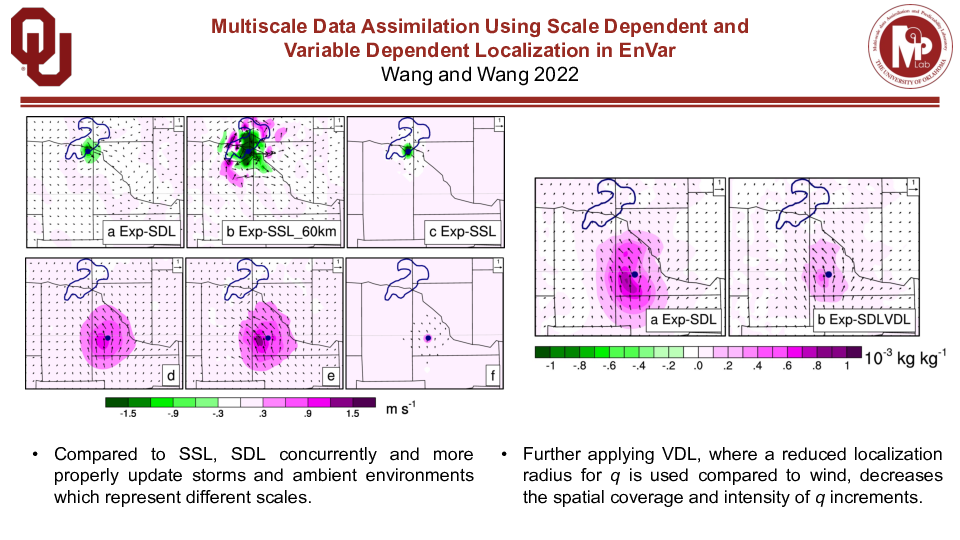
[Advancement of hybrid EnVar theory]
From Wang et al. (2007)
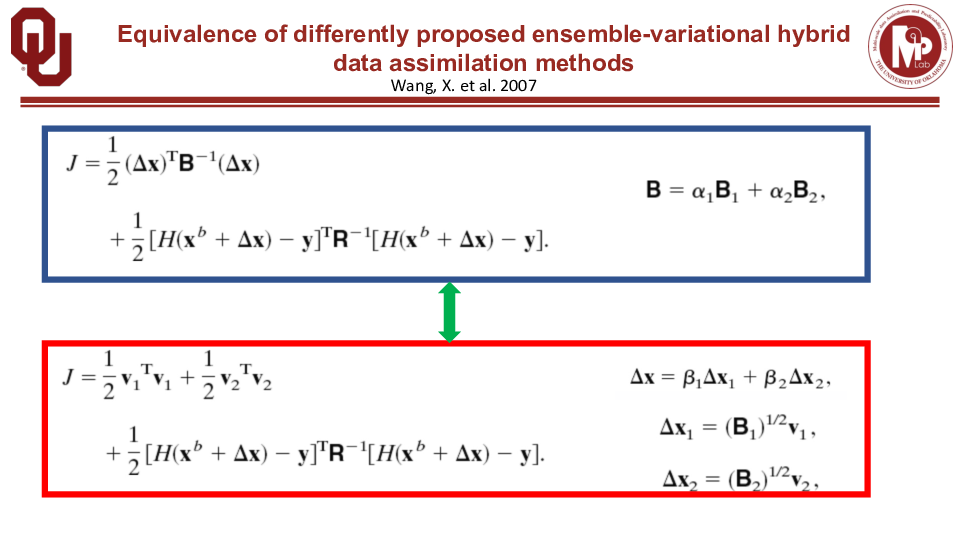